Google’s Art Project Magenta managed to create its first melody through the machine learning device. The result was unveiled on Wednesday and was built on top of Google’s TensorFlow, the company’s open-source Artificial Intelligence (AI) engine.
The result was a 90-second piano melody created by Magenta, which algorithm started with only four notes and kept learning until its final piece. It appears to be a successful attempt for Google’s latest project. As one of the Magenta’s primary goals was to design algorithms that learn how to generate art and music, according to the project’s description.
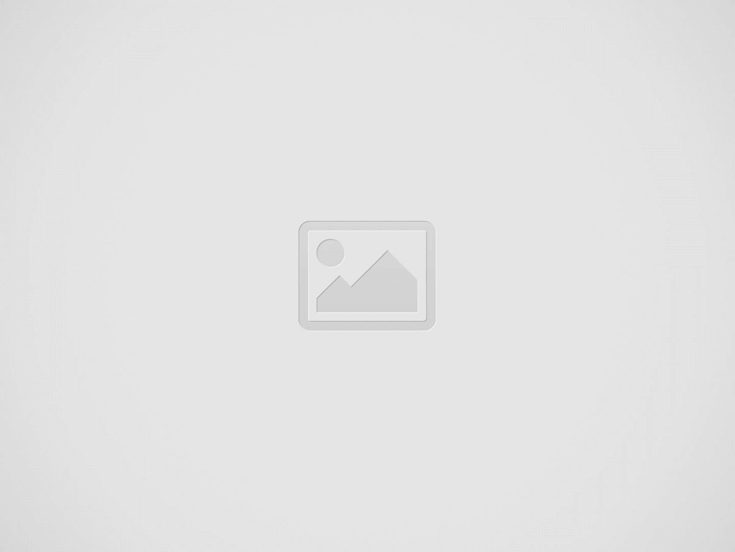
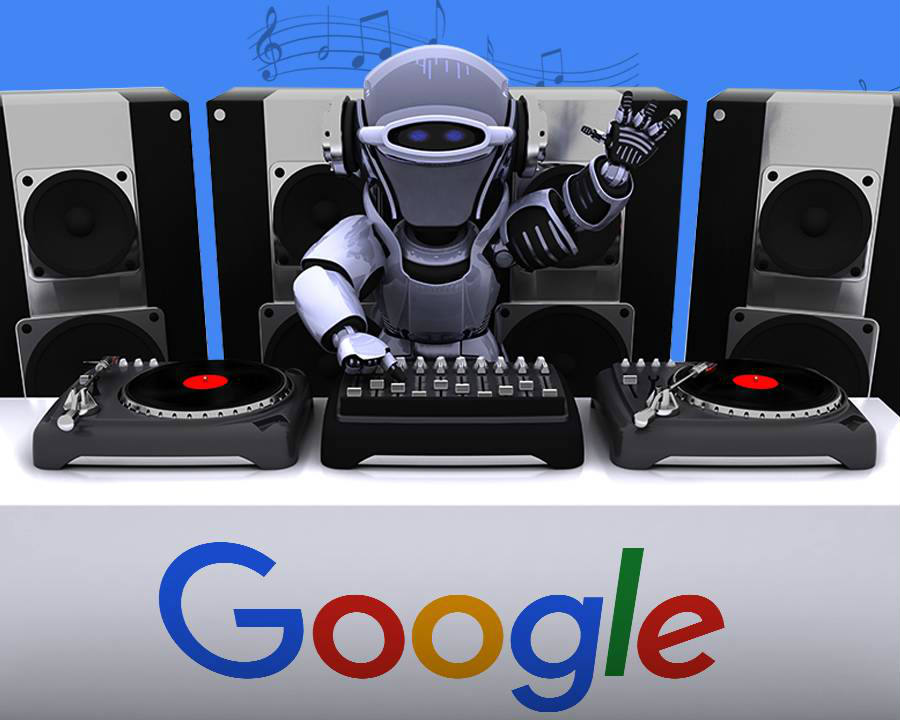
“There has been a lot of great work in image generation from neural networks, such as DeepDream from A. Mordvintsev et al. at Google and Neural Style Transfer from L. Gatys et al. at U. Tübingen,” wrote Magenta’s scientist Douglas Eck in a blog post presenting the project. “We believe this area is in its infancy, and expect to see fast progress here.”
However, besides the already-achieved goal, Magenta has two others in mind. According to Eck, the first one it is a research project to advance the state of the art in machine intelligence for music and art generation.
Bringing the artistic side of machines
Machine learning has already been used extensively to understand content, as in speech recognition or translation, Eck commented. With Magenta, the team wants to explore ‘the other side’ by developing the algorithms that can learn how to generate art and music and potentially creating compelling and artistic content on their own, he added.
As for the second one, Eck said that Magenta is also an attempt to build a community of artists, coders, and machine learning researchers. Magenta’s future is headed for the team to build an open-source infrastructure around TensorFlow for making art and music. Developers say they will release their models and tools in open source on GitHub as reported by Billboard.
Building a community
Magenta is aiming to create a community where interesting people is there to help out. It is based as well on constant feedback from its users so the project will know exactly what to fix and when, and even do it together is the user wants.
However, Magenta’s evaluating process could be the most difficult thing due to not all the pieces from the AI device, or its contributors will be the best ones in the area.The project has some options to determine which one out of the 20 different music generation models available in open source is the best.
One option is to compare model output to training data by measuring likelihood, although this may not work very well according to Eck. It is easy to generate outputs in that are close regarding the likelihood, but far in terms of appeals and vice versa, he said. The other one is to answer the evaluation questions directly from the artists and musicians, as well from Magenta’s viewers and listeners.
Source: Magenta